Python Set Operations Explained: From Theory to Real-Time Applications
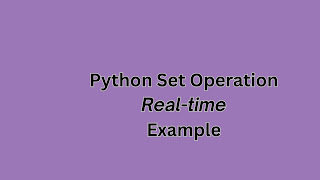
A set in Python is an unordered collection of unique elements. It is useful when storing distinct values and performing operations like union, intersection, or difference. Real-Time Example: Removing Duplicate Customer Emails in a Marketing Campaign Imagine you are working on an email marketing campaign for your company. You have a list of customer emails, but some are duplicated. Using a set , you can remove duplicates efficiently before sending emails. Code Example: # List of customer emails (some duplicates) customer_emails = [ "alice@example.com" , "bob@example.com" , "charlie@example.com" , "alice@example.com" , "david@example.com" , "bob@example.com" ] # Convert list to a set to remove duplicates unique_emails = set (customer_emails) # Convert back to a list (if needed) unique_email_list = list (unique_emails) # Print the unique emails print ( "Unique customer emails:" , unique_email_list) Ou...
Comments
Post a Comment
Thanks for your message. We will get back you.