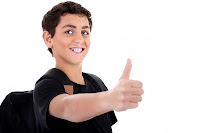 |
#The best visualization tool Tableau for Software Developers: |
Why Tableau:
Companies that have invested millions of dollars in
BI systems are using spreadsheets for data analysis and reporting.
When BI system reports are received, traditional tools often employ inappropriate visualization methods. People want to make informed decisions with reliable information. They need timely reports that present the evidence to support their decisions. They want to connect with a variety of datasources, and they don't know the best ways to visualize data. Ideally, the tool used should automatically present the information using the best practices.
3 Kinds of Data
Known Data (type 1)
Encompassed in daily, weekly, and monthly reports that are used for monitoring activity, these reports provide the basic context used to inform discussion and frame questions. Type 1 reports aren't intended to answer questions. Their purpose is to provide visibility of operations.
Data YOU Know YOU need to Know (type 2)
Once patterns and outliers emerge in type 1 data the question that naturally follows is: Why is this happening? People need to understand the cause of the outliers so that action can be taken. Traditional reporting tools provide a good framework to answer this type of query as long as the question is anticipated in the design of the report.
Data YOU don't Know YOU need to Know (type 3)
By interacting with data in real-time while using appropriate visual analytics, Tableau provides the possibility of seeing patterns and outliers that are not visible in type 1 and type 2 reports. The process of interacting with granular data yields different questions that can lead to new actionable insights. Software that enables quick-iterative analysis and reporting is becoming a necessary element of effective business information systems.
Distributing type 1 reports in a timely manner is important, but speed in the design and build stage of type 1 reports is also important when a new type 1 report is created. To effectively enable type 2 and 3 analyses the reporting tool must adapt quickly to ad hoc queries and present the data in intuitively understandable ways.
Comments
Post a Comment
Thanks for your message. We will get back you.